Lifelong learning is now something that is expected of us everyday and we are facing major challenges with the rapid development of new technologies such as 5G, Artificial Intelligence (AI) and Robotic Process Automation (RPA). Now it is a matter of equipping the audit department with the appropriate competencies at an early stage in order to be prepared for the future. For this reason, we are now going to take a look at the risks involved in using AI to support decision making. In this context, we will also discuss the framework recently published by The Institute of Internal Auditors (IIA) for the auditing of AI.
AI Framework – too big to fail?
In order to shed light on the use of Artificial Intelligence (AI) within a company with regard to aspects of compliance, it is always helpful to look to standards and frameworks as a point of orientation.
Since AI is only just beginning to take hold in companies, frameworks for compliance or audit aspects regarding AI are still under development. The “Artificial Intelligence Auditing Framework” of the Institute of Internal Auditors (IIA) can be regarded as the first such framework [1]:
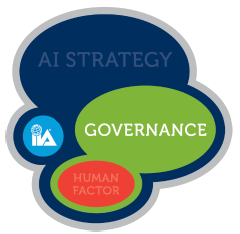
This is subdivided into the following areas:
- AI Strategy and
- AI Governance
- human factors
As usual with the IIA’s frameworks , the AI Framework also defines Control Objectives, as well as Activities and Procedures. The basic information on the framework can be found here.
An evaluation of the AI Framework leads to the following points of criticism:
- The AI Framework goes “top-down” and starts with the assessment of the company’s AI strategy. At present, however, it cannot be assumed that many companies have formulated something that could be considered to be an AI strategy even in the broad sense, unless you look at companies as Amazon, Facebook or Google. If there is a lack of an AI strategy, then the AI Framework may appear to be a kind of “headless” orientation framework.
- The situation is similar with “AI Governance”. The fact that there are explicit guidelines and policies for AI-controlled processes in companies is probably still the exception at the time of writing. Uniform governance for AIs is probably only something that can really be expected in the future.
- The AI Framework also takes up the topic of “Cyber Resilience”. This topic really belongs more to the field of IT security and the references to AI are rather indirect.
The explanations on Data Architecture & Infrastructure and on the topic of data quality are important and give the user concrete guidance on the use of AI within the company.
Overall, the AI Framework for practical use in the company on the basis of “being prepared for tomorrow today” still appears to be somewhat abstract and not necessarily that practical.
What can go wrong?
At this point, I recommend taking a different approach to the AI Framework and following a “bottom-up” approach. The question we should be asking is: What can go wrong when using AI? To answer this, we need to attempt to carry out a compliance-oriented evaluation.
In what follows, we describe three stylized negative phenomena connected with the use of AI: the rule blackbox, out-of-context bias and feedback loop bias.
Rule Blackbox
The rule blackbox is a problem of advanced AI methods. The problem is that an AI can make good decisions, but this decision is almost impossible for a human being to comprehend and explain.[2] “Explainability” is therefore a separate area of research within AI, the aim of which is to make AI decisions comprehensible for humans.
Results that are difficult to understand can also arise if the training data contain (insignificant) features on which the AI (incorrectly) orients its decisions. This is problematic because insignificant characteristics correlate with characteristics in the training data set, which are significant, but more difficult to process, whereby this correlation does not necessarily predominate in the (unknown) population.
This phenomenon can even be exploited, in that a backdoor is put in the training data to deliberately deceive the AI during the learning process.[3] For example, it has been reported to be possible to provide a backdoor in the form of a given pair of glasses so that the AI learned during facial recognition instead of dealing with the recognition of the actual face. People with other glasses were not recognized, although it might have been possible to do so. The AI focused on the glasses instead of the faces.
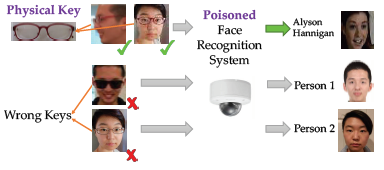
Figure: A pair of glasses as a “backdoor” feature, wrongly used as a point of orientation by the AI[4]
Unwanted results can still be produced by an AI if the training data contains data for which certain characteristics have very asymmetric expressions. In the COMPAS system (Correctional Offender Management Profiling for Alternative Sanctions), for example, which gives an assessment of risk values for offenders, assessments were made on the basis of ethnic origin, since certain ethnic groups were disproportionately represented in the training sample.[4]
Similar effects can also occur, for example, with the automatic (pre-)evaluation of persons as part of application procedures with regard to characteristics such as age or gender.
Out-Of-Context Bias
A further distortion of AI results may occur if the AI is trained in a particular context but then operated in a different or altered context. To avoid out-of-context bias, the framework and context should be kept constant. Thus, it was possible to show that an AI in the form of a neural network could easily be deceived by changing the angle of objects on images.[5] The AI delivered bizarre results regarding object recognition.
Feedback-Loop Bias
A feedback loop bias is something like a “self-fulfilling prophecy”. This distortion occurs when, based on the analysis results of an AI, action is taken in a certain way and this action in turn influences relevant characteristics for the AI (in a certain direction). The action thus changes the characteristic values and cements conclusions. The AI feeds itself with its own forecasts. One example is “PredPol USA – Predictive Policing”[6] for planning police patrols. This leads to a feedback loop:
Increased police patrols
=> more arrests
=> increased police presence
=> increased estimation of criminality.
The conclusion is then obvious:
more police presence => more crime.
In the next blog post, we will show three concrete steps that can be taken to assess AI-based decisions.
Sources
[1] vgl. Darpa: Explainable Artificial Intelligence (XAI): https://www.darpa.mil/program/explainable-artificial-intelligence
[2] vgl. Chen, Liu, Li, Lu, Song: Targeted Backdoor Attacks on Deep LearningSystems Using Data Poisoning, https://arxiv.org/pdf/1712.05526.pdf
[3] Institute of Internal Auditors (2019): GLOBAL PERSPECTIVES AND INSIGHTS – The IIA’s Artificial Intelligence Auditing Framework Practical Applications, Part A
[4] vgl. Larson, Mattu, Kirchner, Angwin (2016): How We Analyzed the COMPAS Recidivism Algorithm, https://www.propublica.org/article/how-we-analyzed-the-compas-recidivism-algorithm
[5] vgl. Alcorn, Li, Gong, Wang, Mai, Ku, Nguyen (2019): Strike (with) a Pose: Neural Networks Are Easily Fooled by Strange Poses of Familiar Objects, https://arxiv.org/pdf/1811.11553.pdf
[6] vgl. PredPol USA – Predictive Policing, https://www.predpol.com